Table of Contents
Beyond Rain Checks: AI Tackles Earth’s Fury
Remember checking your weather app this morning, wondering if you needed an umbrella? It’s a routine part of modern life. But what if that same predictive power, amplified exponentially, could warn us not just about a passing shower, but about nature’s most destructive forces? Forget just guessing the chance of rain; Artificial Intelligence is stepping into the high-stakes arena of predicting actual natural disasters.
It sounds almost like science fiction, doesn’t it? The idea that complex algorithms could anticipate the earth-shattering power of an earthquake, the swirling chaos of a hurricane, or the devastating surge of a flood. Yet, this isn’t a far-off dream. Right now, AI systems are being developed and deployed, learning to decipher the planet’s subtle warnings in ways humans never could.
The potential is staggering: reliable warnings delivered days, not just hours, before disaster strikes. That’s precious time – time to evacuate, secure property, and ultimately, save countless lives. It’s a technological leap that truly feels world-changing.
Speaking of mind-blowing tech making waves, we whipped up a quick visual take on this very idea. See AI disaster prediction in action (well, animated action!):
How Does AI Peer into Nature’s Playbook?
So, how exactly does this digital oracle work its magic? It’s not about crystal balls; it’s about data – massive amounts of it. AI, particularly machine learning (ML) and deep learning models, thrives on identifying patterns hidden within complex datasets. For natural disaster prediction, this involves:
- Crunching Historical Data: AI analyzes decades, even centuries, of records about past disasters – where they struck, their intensity, preceding conditions, and impacts. This helps establish baseline patterns and identify recurring indicators.
- Real-time Sensor Networks: Data streams in constantly from seismometers (detecting ground motion), GPS stations (tracking tectonic plate shifts), weather buoys (measuring ocean conditions), river gauges (monitoring water levels), and atmospheric sensors (tracking pressure, temperature, humidity).
- Satellite and Radar Imagery: Eyes in the sky provide invaluable visuals. AI algorithms scan satellite images to monitor cloud formations for hurricanes, track ground deformation for earthquakes or volcanic activity, assess vegetation dryness for wildfire risk, and map potential floodplains.
- Atmospheric and Oceanic Modeling: Complex simulations of weather systems and ocean currents are fed into AI models, which can then refine predictions about storm paths, intensity changes, and storm surges.
- Even Social Media & News: Sometimes, early reports or unusual activity patterns surface on social media or local news. AI can be trained to sift through this unstructured data to potentially corroborate or even precede official sensor alerts (though this requires careful filtering).
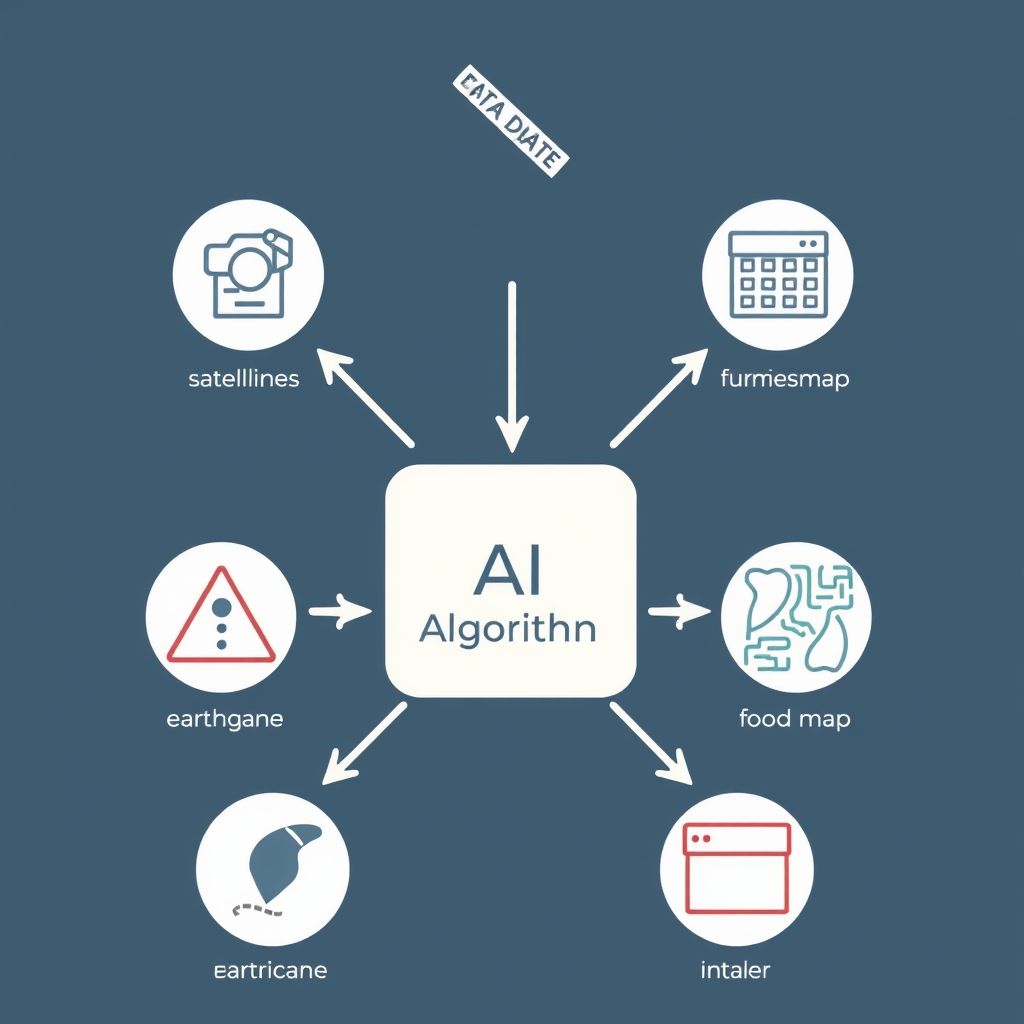
Machine learning algorithms are trained on this diverse data cocktail. They learn to spot subtle anomalies, correlations, and precursor signals that might escape human analysis. For instance, an algorithm might detect a specific pattern of micro-tremors preceding a larger earthquake or identify unique atmospheric conditions that historically lead to rapid hurricane intensification.
AI vs. The Elements: Specific Applications
Let’s break down how AI is being applied to different types of natural disasters:
Earthquake Forecasting: The Tremor Predictor
Predicting the exact time and place of a major earthquake remains one of science’s greatest challenges. However, AI is making inroads:
- Analyzing Seismic Waves: Deep learning models can analyze the subtle characteristics of P-waves (the faster, initial seismic waves) to estimate the magnitude and potential impact of an earthquake seconds before the more destructive S-waves arrive. This allows for ultra-short-term warnings – enough time to trigger automated shutdowns of gas lines or alert surgeons in operating rooms.
- Aftershock Prediction: AI is proving quite effective at predicting the likelihood and location of aftershocks following a major quake, which is crucial for rescue and recovery efforts.
- Pattern Recognition: ML algorithms sift through vast datasets of historical seismic activity and ground deformation (measured by GPS) to identify potential long-term risk patterns or subtle precursory signals, although reliable long-term prediction is still largely experimental.
Disclaimer: While promising, AI for earthquake prediction, especially long-term forecasting, is still an active area of research. The chaotic nature of fault dynamics makes precise prediction incredibly difficult.
Hurricane & Cyclone Tracking: Taming the Tempest
Weather agencies already use sophisticated computer models, but AI adds another layer of predictive power:
- Intensity Forecasting: AI models analyze satellite imagery (cloud patterns, eye structure) and atmospheric data to better predict if a storm will rapidly intensify – a notoriously difficult task for traditional models.
- Path Prediction: By learning from vast amounts of historical storm track data and real-time atmospheric conditions, AI can help refine path predictions, offering more accurate landfall projections.
- Storm Surge & Rainfall Modeling: AI can integrate storm characteristics with coastal topography and hydrological data to predict the extent and depth of potentially deadly storm surges and inland flooding more accurately.
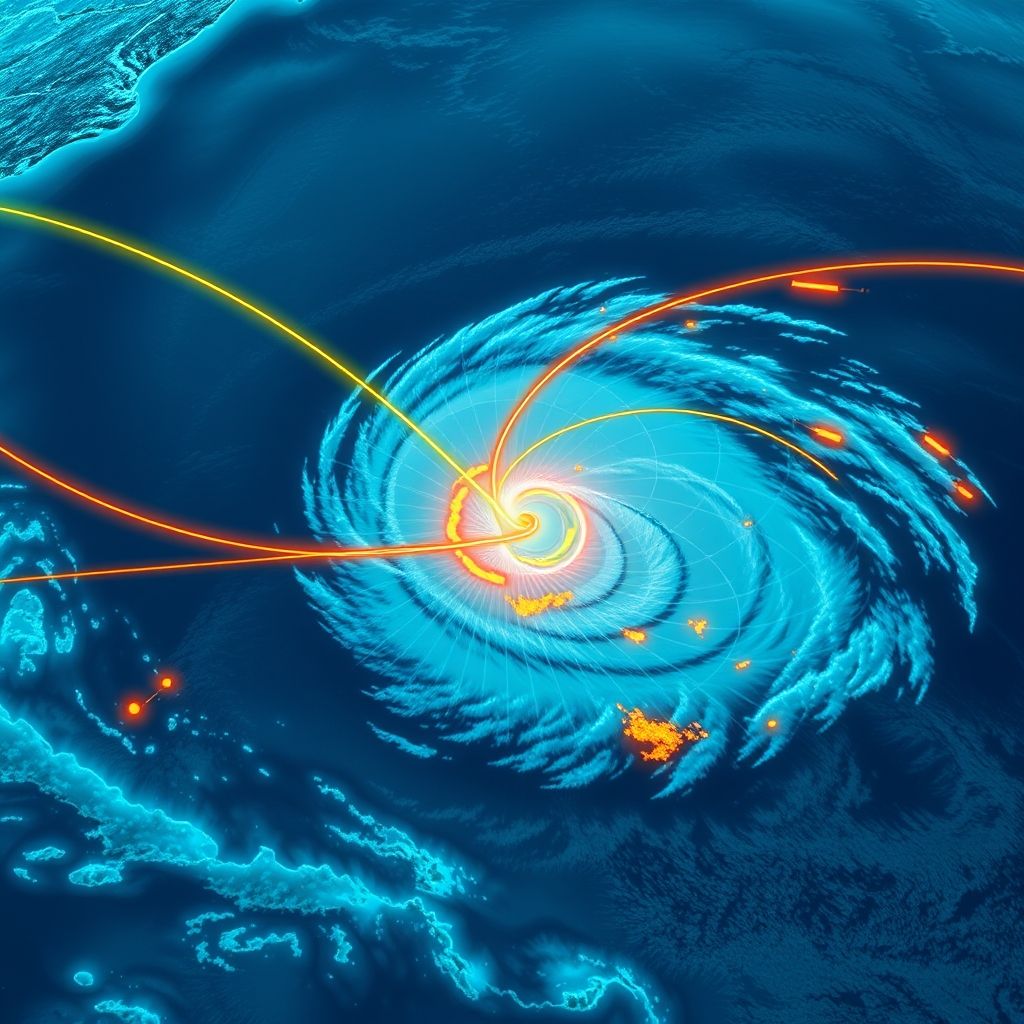
Flood Prediction: Stemming the Tide
Floods can result from heavy rainfall, overflowing rivers, or storm surges. AI helps anticipate them by:
- Analyzing Rainfall Data & Weather Models: Predicting how much rain will fall and where is key. AI improves short-term rainfall forecasts (nowcasting).
- River Flow Monitoring: ML models analyze data from river gauges, upstream conditions, and snowmelt patterns to predict when rivers are likely to crest their banks.
- Inundation Mapping: By combining rainfall predictions, river flow data, and detailed terrain maps (LIDAR), AI can generate highly localized maps showing which specific areas are likely to flood and to what depth.
Wildfire Management: Fighting Fire with Data
AI assists firefighters and authorities in several ways:
- Early Detection: Drones and satellites equipped with thermal cameras and AI image recognition can detect new wildfires much earlier than traditional watchtowers, especially in remote areas.
- Spread Prediction: AI models simulate how a fire might spread based on fuel type (vegetation maps), topography, and real-time weather conditions (wind speed, humidity), helping allocate resources effectively.
- Risk Assessment: Analyzing historical fire data, climate patterns, and vegetation conditions helps identify areas at high risk *before* a fire starts.
The Real Payoff: Earlier Warnings, Safer Communities
The ultimate goal of AI in disaster prediction isn’t just academic curiosity; it’s about tangible benefits:
- Increased Warning Lead Time: This is the game-changer. Getting warnings days or even just hours earlier allows for organized evacuations, reducing loss of life dramatically.
- Improved Resource Allocation: Knowing where and when a disaster is likely to hit allows emergency services to pre-position personnel, equipment, and supplies more effectively.
- Enhanced Situational Awareness: During an event, AI can process real-time data feeds to give responders a clearer picture of the evolving situation, helping prioritize rescue efforts.
- Infrastructure Protection: Early warnings can allow operators time to shut down critical infrastructure (power grids, transportation networks) safely or reinforce vulnerable structures.
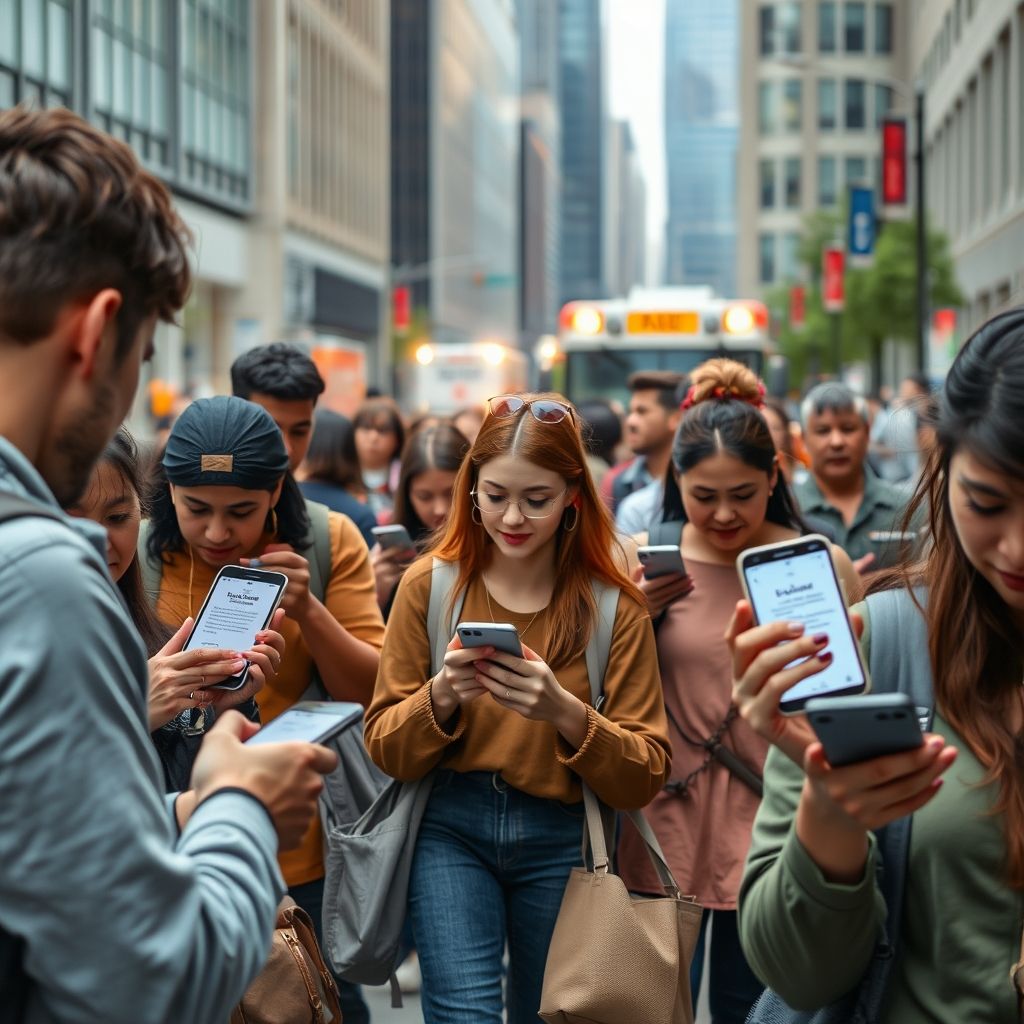
Navigating the Challenges: Hurdles on the Path Forward
Despite the immense potential, using AI for disaster prediction isn’t without its challenges:
- Data Dependency: AI models are only as good as the data they’re trained on. Gaps in historical data, poor sensor coverage, or low-quality data can hinder accuracy.
- Computational Cost: Training complex deep learning models requires significant computing power.
- The ‘Black Box’ Problem: Understanding *why* an AI made a specific prediction can sometimes be difficult, making it harder to trust or troubleshoot.
- Nature’s Unpredictability: Natural systems are inherently chaotic. AI improves probabilities, but it cannot eliminate uncertainty entirely. Unexpected factors can always arise.
- False Alarms & Complacency: Striking the right balance is crucial. Too many false alarms can lead to public distrust or complacency (‘crying wolf’).
- Integration & Implementation: Developing the AI is one thing; integrating it seamlessly into existing emergency management workflows and communication systems is another challenge.
The Future is Forecast: What’s Next?
The field of AI-driven disaster prediction is evolving rapidly. We can expect:
- More Sophisticated Algorithms: Continued advancements in AI research will lead to even more accurate and nuanced predictive models.
- Integration with IoT: Expect wider sensor networks (Internet of Things devices) providing even more granular real-time data.
- Hybrid Approaches: Combining the strengths of AI with physics-based simulation models will likely yield the best results.
- Improved Communication: Better ways of disseminating warnings generated by AI systems to the public and emergency responders.
While AI won’t stop natural disasters from happening, it represents a monumental leap forward in our ability to anticipate them and mitigate their devastating impacts. It’s transforming disaster management from a purely reactive discipline to an increasingly proactive one, offering humanity a powerful new tool in adapting to our dynamic planet.
Frequently Asked Questions (FAQs)
Q1: Can AI predict earthquakes with 100% accuracy?
A1: No. Currently, AI cannot predict the exact time, location, and magnitude of major earthquakes with high certainty long in advance. It’s better at short-term warnings (seconds/minutes) based on initial wave detection and predicting aftershock patterns. Long-term forecasting remains highly experimental.
Q2: How is AI different from traditional weather forecasting models?
A2: Traditional models are largely physics-based, simulating atmospheric processes. AI, particularly machine learning, excels at identifying complex patterns and correlations in vast historical and real-time data that physics-based models might miss. Often, the best results come from combining both approaches.
Q3: What are the biggest data sources for AI disaster prediction?
A3: Key sources include satellite imagery, weather station data, seismic sensor networks, GPS data, river gauges, ocean buoys, radar systems, historical disaster records, and topographic maps.
Q4: Are there ethical concerns with using AI for disaster warnings?
A4: Yes. Key concerns include the potential for false alarms causing panic or complacency, ensuring equitable access to warnings across different communities, data privacy related to sensor networks, and transparency in how AI models arrive at their predictions.
Q5: How far away are we from widespread use of AI disaster warnings?
A5: Elements are already in use or development. AI is increasingly integrated into hurricane intensity forecasting and flood mapping. Early warning systems for earthquakes based on AI analysis of initial waves exist in some regions. Widespread, highly reliable prediction across all disaster types is still evolving but progressing rapidly.